
Health. “The enjoyment of the highest attainable standard of health is one of the fundamental rights of every human being without distinction of race, religion, political belief, economic or social condition” as WHO puts it. IBM believes that AI and machine learning are changing how we deliver healthcare. Healthcare providers have aggregated a vast amount of data in the form of health records, images, population data, claims data and clinical trial data. AI technologies are suitable to analyse this data to uncover patterns and insights humans cannot find.
Thomas Davenport and Ravi Kalakota (2019) suggest that the healthcare industry will increasingly apply AI within the field because of the complexity and rise of data. Several categories of applications include diagnosis and treatment recommendations, patient engagement and adherence, and administrative activities.
There are several benefits of AI in healthcare:
Providing user-centric experiences
Improving efficiency in operations
Connecting disparate healthcare data
1. Types of AI applicable and regulations in AI in healthcare:
Thomas Davenport and Ravi Kalakota (2019) assert we can apply machine learning in precision medicine. It helps anticipate what treatment protocols will likely succeed on a patient based on various patient attributes and their treatment contexts. It mainly requires supervised learning. We may employ deep learning to recognise potentially cancerous lesions in radiology images.
Natural language processing (NLP) has applications frequently in creating, understanding and classifying clinical documentation and published research. NLP systems may analyse unstructured clinical notes on patients, prepare reports, transcribe patient interactions and conduct conversational AI.
Rule-based expert systems can be widely helpful for "clinical decision support" purposes in the last couple of decades. They require human experts and knowledge engineers to develop a rules series in a particular knowledge domain. We are slowly replacing them with techniques based on data and machine learning algorithms.
Physical robots perform pre-defined tasks like lifting, repositioning, welding or assembling objects. They have become more collaborative with humans and more trainable. Surgical robots provide surgeons with unprecedented capabilities to see and create precise and minimally invasive incisions, stitch wounds and so forth in gynecologic surgery, prostate surgery, and head and neck surgery.
Robotic process automation (RPA) performs structured digital tasks for administrative purposes. We use it for repetitive tasks like prior authorisation, updating patient records or billing. When we combine it with other technologies like image recognition, we can use them to extract data into transactional systems. In the future, we can merge and integrate these technologies. Synthesised solutions will be more feasible.
There is a lot of attention for IBM’s Watson in the media since it focuses on precision medication, particularly cancer diagnosis and treatment. It uses a combination of machine learning and NLP capabilities. However, teaching Watson how to address particular types of cancer and integrate them into care processes and systems was challenging. Most people feel that Watson APIs are technically capable, but taking on cancer treatment was an overly ambitious objective.
AI implementation challenges might trouble many healthcare organisations. Though rule-based systems integrated within EHR systems are commonplace, including at the NHS, they lack the precision of more algorithmic systems based on machine learning. These rule-based clinical decision support systems are hard to maintain since medical knowledge changes. They cannot handle the explosion of data and knowledge based on genomic, proteomic, metabolic and other "omic-based" approaches to care. The situation is beginning to change. However, it predominantly exists in research labs and tech companies than clinical practice. Many clinical findings depend on radiological image analysis, though some involve other types of images, including retina scanning or genomic-based precision medicine. These rely on statically-based machine learning models. They are propelling in an era of evidence-and probability-based medicine, which we generally regard as positive, but still possess many challenges in medical ethics and patient/clinic relationships.
Technology firms and startups are also working assiduously on the same issues.
Both providers and payers for care use "population health" machine learning models to predict the at-risk population of particular diseases or accidents. However, they sometimes lack all the relevant data that might add predictive capability, including patient socio-economic status, even when these models can effectively predict.
We have long seen patient engagement and adherence as the "last mile" problem of healthcare – the final barrier between ineffective and good health outcomes. The more patients proactively support their well-being and care, the better the results. We increasingly address these factors with big data and AI. We increasingly focus on machine learning and business rules engines to drive nuanced interventions along the care continuum. Messaging alerts and relevant, targeted content provoking actions at crucial moments is a promising research field. Another increasing emphasis is effectively designing the “choice architecture” to nudge patient behaviours more anticipatorily based on real-world evidence.
The use of AI in administrative applications is probably less revolutionary than patient care. However, it can still be substantially efficient. The technology that is most likely to be relevant to this objective is RPA. It is helpful for various applications in healthcare, including claims processing, clinical documentation, revenue cycle management and medical records management. There are NLP-based applications for patient interaction, mental health and wellness, and telehealth that are effective for simple transactions like refilling a prescription or making appointments. However, it may concern some patients about revealing confidential information, discussing complex health conditions and poor usability. Machine learning can also be helpful in claims and payment administration.
According to the Chinese Journal of Health Policy, The US Food and Drug Administration (FDA) began to establish a regulatory computer-aided identification system in 1998. CAD, an imaging system currently approved to use in breast screening, can enhance radiologists' readings. In 2012, the FDA published a relatively comprehensible selection of review metrics for software that integrates machine learning algorithms, including algorithm design, features, models, data sets used to train and test the algorithms, and the "healthiness" of the test data used. In 2015, the FDA published a metrics collection to review software that integrates machine learning algorithms. In 2015, the FDA issued a letter defining controlled cardiac ablation catheter remote control systems as Class II, a medium risk. The FDA is committed to accelerating patient access to innovative medical devices that meet needs by shifting some of the evidence that needs gathering during the product development process to after the technology is available on the market. Currently, it classifies most medical devices in one of two ways: high-risk devices (also known as Class III products) generally evaluated through a premarket approval (PMA), where the manufacturer must conduct at least one clinical study and submit relevant data to the FDA. They review medium- and low-risk products (Class I and Class II products), usually to assess whether they are comparable to devices already on the market, which only occasionally require clinical trial data. The FDA aims to complete its review of PMA applications within 180 days (or 320 days if the assessment requires a federal advisory committee). In 2016, the FDA issued three specifications pivotal to the future of medical innovation: (1) a legal specification for low-risk general health products; (2) a legal specification to provide practical evidence-based support for medical device regulatory decisions; and (3) an adaptive design specification for clinical trials for medical device access. These three specifications give framework guidance recommendations for future AI innovation and creativity in healthcare. In 2017, the FDA formally authorised Dr Bakul Patel to form a new division dedicated to digital health and AI technology review, including 13 software engineers and developers, AI technology and cloud computing experts, and others. The department is responsible for preparing specifications and standards for the FDA to begin reviewing the growing influx of AI products, medical and health devices, instruments or medical software with machine learning capabilities, etc. They will re-plan which path of regulation and approval we should use for intelligent medical robots and medical devices with machine learning capabilities.
In February 2017, in China, the National Health and Family Planning Commission revised 15 medical technology management regulations that "restrict clinical application". These include technical management specifications and quality control indicators for AI-assisted diagnosis. In October 2017, the General Office of the CPC Central Committee and the General Office of the State Council issued Opinions on Deepening the Reform of the Review and Approval System to Encourage Innovation in Pharmaceuticals and Medical Devices, stating that they should improve and implement the drug trial data protection system, with a certain period of data protection; medical device marketing licensees should assume full legal responsibility; the technical review system needed improvements, and there should be drug review teams responsible for new drugs. The State Food and Drug Administration (SFDA) of China has also established a medical device review team to review innovative medical devices. The State Food and Drug Administration (CFDA) of China also has a selection of regulatory measures involving decision support and diagnostic aids for medical software as Class III medical devices. The Guidelines for Technical Review of Medical Device Software Registration promulgated in 2015 require software description documents to include basic information, implementation process and core algorithms. In August 2017, they issued an opinion draft of the Drug Data Management Specification to regulate data management for all activities in the product life cycle, requiring senior management to be ultimately responsible for the drug data reliability. In September 2017, the CFDA stipulated that applicants should submit applications for classification definition through the Classification Definition Information System of the General Administration's Medical Device Standards Management Centre. In general, China has some policies that respond to the arrival of AI. However, there is not yet a new department dedicated to reviewing digital healthcare and AI technologies, while the US has already started setting up a relevant department. Looking ahead to the future development of AI and its broader impact on society, establishing a dedicated management department is urgent for many countries.
2. Challenges in healthcare datasets and how to solve them
Mary Sowjanya & Owk Mrudula (2022) suggests that datasets in healthcare may be commonly imbalanced. KD Nuggets proposed 7 techniques to handle imbalanced data: using the right evaluation metrics, resampling the training set by undersampling or oversampling, using K-fold cross-validation in the right way, ensemble different resampled datasets, resampling with different ratios, clustering the abundant class, designing your models.
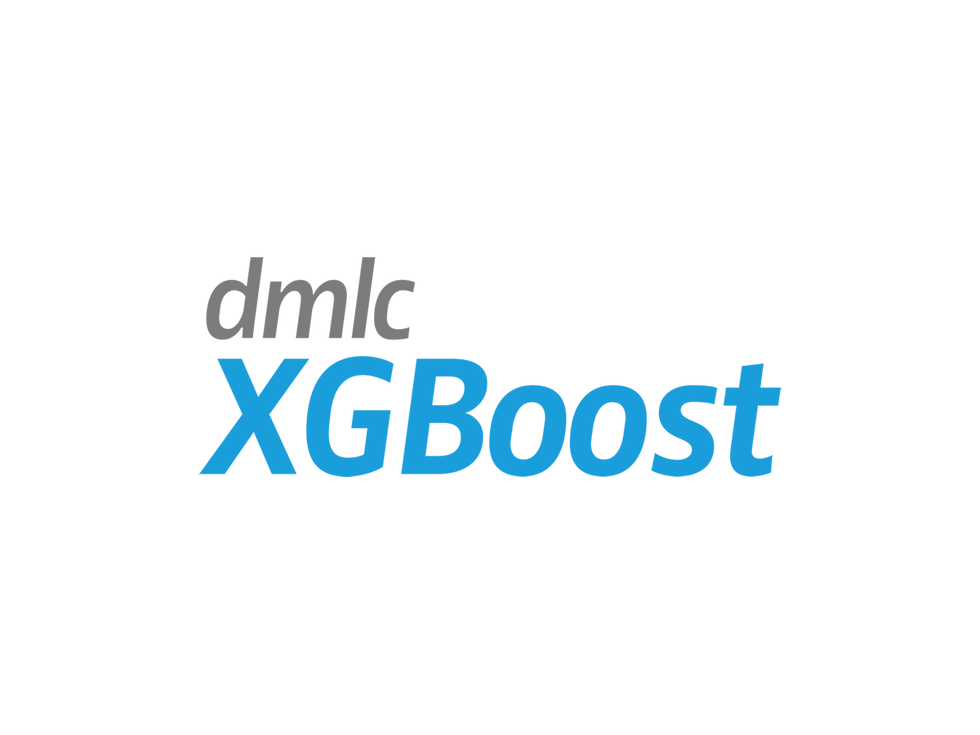
Sampling techniques like Synthetic Minority Oversampling Technique (SMOTE) can only provide moderate accuracy in a proper analysis of cumulative datasets. They proposed two different methods: distance-based SMOTE and Bi-phasic SMOTE. The D-SMOTE generates new examples rather than duplicating the minority class examples. The technique generates new “synthetic” samples in the vicinity of minority classes. It consists of adjusting the distance between the ones based on the hierarchy of classes. However, it introduces additional noise in the form of unimportant variables while creating new synthetic ones. BP-SMOTE is another technique to use. It involves two levels: SMOTE and then instance selection. In phase 1: we use the original SMOTE to maximise the minority cases in the original data. In phase 2: in instance selection, the representative instances are chosen with greedy selection as the final training dataset. BP-SMOTE changes the functional vectors of the sampled instances by multiplying a parameter to adjust the data set feature spaces but not to modify the data space, helping the minority class to divide their area and enter the border of the majority class. We can use a stacking approach to increase accuracy, including Stack Convolutional Neural Network (Stacked CNN) and Stacked Recurrent Neural Network (Stacked RNN). Stacked CNN will also be highly accurate since different CNN sub-models learn non-linear discriminative features and semantic representation at different levels of abstraction. Stacked RNN is for time-series data.

Working in systems biology, we can store data in graphs to connect complex data. Graph algorithms traverse the graph and identify a desired triangular node pattern linking the three classes of data together. Graph analytics can find relevant nodes in the desired triangular relationship and employ a metric to gauge the associated strength between each node in each triangle. Santiago Timón-Reina, Mariano Rincón and Rafael Martínez-Tomás (2021) state that the biomedical domain is a complex arena studied in many different sub-domains inherently related and connected. Moreover, the large amount of data in the ‘omics’ era eventuates in large graphs difficult to manage without a database optimised for the task. Scenarios with a substantial volume of complex relationships may benefit from GDBMSs (Graph Database Management Systems) since graphs give us more natural modelling of many-to-many relationships, graph-oriented query languages supply more intuitive means for writing complex network traversal and graph algorithm queries than table-oriented ones like SQL, the schema-less/optional grants flexibility, and most frequently GDBMSs display higher performance for relationship-centric searches, like path traversals. These features convey several benefits for the biomedical domain, such as easing the communication between domain experts, providing tools for discovering entities/clusters/patterns within the graph structure and facilitating data integration tasks. These graph databases have “minute to milliseconds” performance while dramatically accelerating development cycles, having extreme business responsiveness and being enterprise-ready.
Missing data in healthcare is commonplace. We need to know why they are missing in the place to have proper ways of dealing. According to Columbia University, we may consider four general “missingness mechanisms”:
Missingness completely at random: the probability of missingness is the same for all units. Removing cases with missing data occurring this way does not bias our inferences.
Missingness at random: most missingness is not completely at random as observed in the data. A more general assumption is that the probability a variable is missing depends only on available information. We may reasonably model this process as a logistic regression where the outcome variable equals 1 for observed cases and 0 for missing ones. When an outcome variable is missing at random, we may exclude the missing cases as long as the regression controls for all the variables affecting the missingness probability.
Missingness depends on unobserved predictors: if missingness depends on information that we have not recorded and this information predicts the missing values, it is no longer “at random”. We then must explicitly model it, or else we must accept some bias in our inferences.
Missingness depends on the missing value itself: a specifically challenging situation arises when the missingness probability depends on the variable itself. We can model or mitigate censoring (when all samples in a particular population refuse to respond) and related missing-data mechanisms by including more predictors in the missing-data model, thus bringing it closer to missing at random. While we can predict missing values based on other variables in our datasets, this circumstance can be more complex in that the nature of the missing-data mechanism may force these predictive models to extrapolate beyond the range of the observed data.
We generally cannot be sure whether data are missing at random or whether the missingness depends on unobserved predictors or missing data. These potential lurking variables are unobserved, so we cannot strike them out. We then have to make assumptions or check concerning other studies. We try to include as many predictors as possible in a model for the “missing at random” assumption to become logical under normal circumstances.
Many missing data approaches simplify the problem by throwing away data. These might cause biased estimates and estimates with greater standard errors due to reduced sample size. These approaches may include complete-case analysis, available-case analysis and nonresponse weighting.
Another approach is to fill in or impute missing data. Imputation is typically one of the best practices to deal with this problem. Imputation methods keep the full sample size, which can benefit bias reduction and precision. However, they may result in different kinds of biases. We can mitigate these biases. The intuition here is that we have substantial uncertainty about the missing values. However, by opting for a single imputation we pretend that we know the true value with certainty.
3. Future of AI in healthcare
Good outcomes for all people in healthcare require comprehensive regulations, morals and ethics. It does not stop there. Datasets in healthcare deserve attention. Future datasets will need innovative databases, like graph databases. Special care around imbalanced datasets and missing data is highly worthwhile.
References
Comments