Paths to Artificial General Intelligence
- laranguyen811
- Jan 3, 2023
- 7 min read
1. AGI and its current challenges
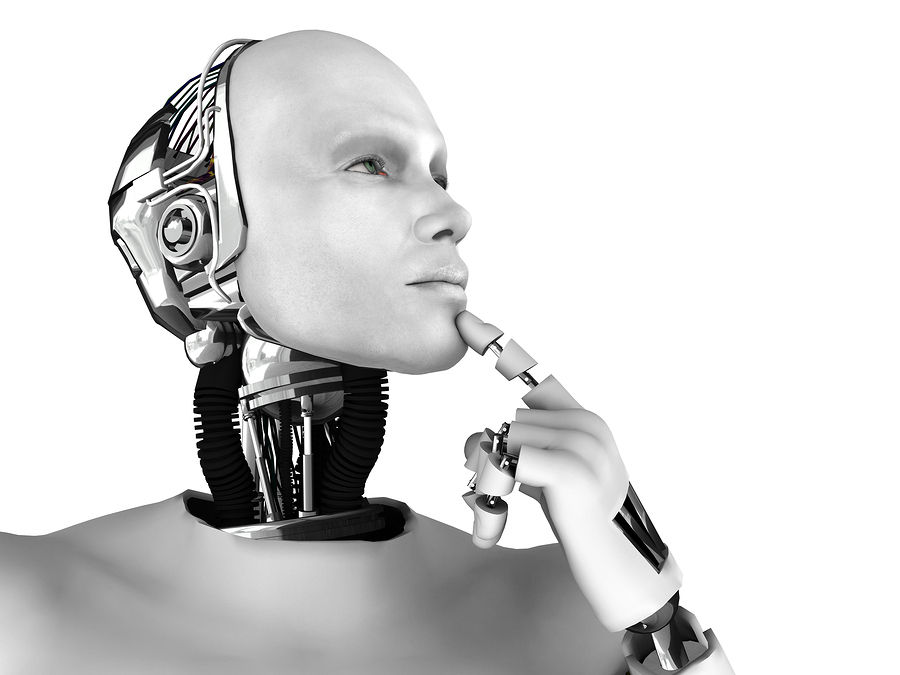
S. Mason Dambrot declares that Artificial General Intelligence (AGI) is a well-researched field focused on building human-analogous AI to perform any human intellectual task flourishingly.
According to Ben Goertzel, we use AGI to describe:
A property of particular systems: “AGI” as the intersection of “artificial” and “general intelligence”.
A system displaying this property: an “AGI” meaning “an AGI system”.
The field of pursuing AGI system creation, and the study of the nature of AGI.
There is a distinction between AGI and “human-level AI”. AGI is a reasonably abstract concept, not intrinsically tied to any particular characteristics of human beings. Several qualities of human general intelligence which are not clear may indeed be prevalent among all powerful AGIs.
What is general intelligence? Ben Goertzel gives us a broad overview of the AGI to explain significant features of general intelligence:
General intelligence includes the ability to achieve various goals and tasks in different contexts and environments.
A general intelligent system can handle problems and situations different from those expected by its creators.
It should be good at generalising the knowledge it has obtained to transfer this knowledge from one problem or context to another.
Arbitrarily general intelligence cannot happen given realistic resource constraints.
Real-world general intelligence is undoubtedly biased toward certain types of goals and environments.
Humans display a higher level of general intelligence than existing AI programs do and also a higher level than other animals.
Humans are unlikely to manifest a maximal level of general intelligence, even relative to the goals and environment to which evolution has enabled them to adapt seemingly.
Various real-world general intelligences may tend to share particular ubiquitous attributes.
Current challenges are:
Due to industry demand, AGI is increasingly addressing specific fields and issues – in the narrow AI realm.
This focus disadvantageously impacts AGI funding and momentum.
It affects the expectations of us developing AGI as anticipated.
Most modern AGI models rely on logic and inner dialogue rather than the affective human cognition foundation, where perception and emotion predate and influence cognitive abilities and decision-making.
The Independent Core Observer Model (ICOM) uses emotion and motivation as humans do rather than having AGI focus on achieving goals, tasks and problems. The ICOM Theory of Consciousness provides AGIs with the most salient but elusive aspect of human awareness – the qualia of consciousness. What is consciousness? Stanford Encyclopedia of Philosophy deems cognitive entities as conscious in several different ways:
Sentience: a sentient creature can sense and respond to its world (Armstrong 1981).
Wakefulness: if it is awake and usually alert.
Self-consciousness: they are not only aware but also aware that they are conscious. We can interpret the level of self-awareness requirement differently with different creatures.
What it is like: a being is conscious if there is “something that it is like” to be that creature, perhaps from a mental or experiential point of view (Nagel, 1974).
Subject of conscious states: we might first define what makes a mental state a conscious mental state and then define being a conscious creature in terms of having such states.
Transitive consciousness: there are related senses in which we describe creatures as conscious of various things.
Perhaps consciousness is different for different entities. What feels like consciousness to us might not be the definition of consciousness for others.
The emotion/perception interaction enables affective information to have immediate and automatic effects without deliberating on the meaning of emotionally evocative stimuli or the consequences of potential actions. We have recently modelled computational empathy in artificial intelligent agents with new advances in neuroscience, psychology and ethology.
2. Paths to AGI

S. MasonDambrot believes that neuromorphic computing emerged in Alan Turing’s paper “Can machines think?”. According to IBM, neuromorphic computing targets addressing the challenges of the next-gen AI by supplying a brain-inspired energy-efficient computing paradigm. Developing AGIs based on AI concepts and code might be incorrect to build human-equivalent functional cognitive structure: the combination of evolutionary neurobiology and self-organised learning.
Counterfactual Quantum Entanglement has given rise to Counterfactual Quantum Communications and Counterfactual Quantum Computation. Counterfactual Quantum Communications counterintuitively enable information exchange without particle interaction. We can accomplish a secret key distribution even though the quantum channel does not allow a particle to carry confidential information to transmit. We can implement the proposed protocol with current technologies – including photonics, and provide practical security advantages by diminishing the possibility that an eavesdropper can directly access the entire quantum system of each signal particle. In counterfactual computation (CFC), inference can achieve certainty while eliminating decoherence-induced errors. We have demonstrated counterfactual quantum computing to achieve high efficiency of up to 85% - well above the 50% limit for standard CF schemes.
Neuromorphic Ecosystems can be dependent on a surprisingly wide range of substrates. Hypothetical Real-Time neuromorphic AGI Ecosystems can be those utilising counterfactual quantum communications to operate in real-time networks, ecosystems based on AGI, and those combining both components.
With the increasing development and diversity of AI and ANNs, an interest in and development of memristors as synaptic transistors, providing key improvements compared with conventional memristors by allowing a large on/off ratio in carbon nanotube channel conductance analog programmability. Tuning carbon nanotube synaptic characteristics optimises the learning rate and attains a higher recognition rate.
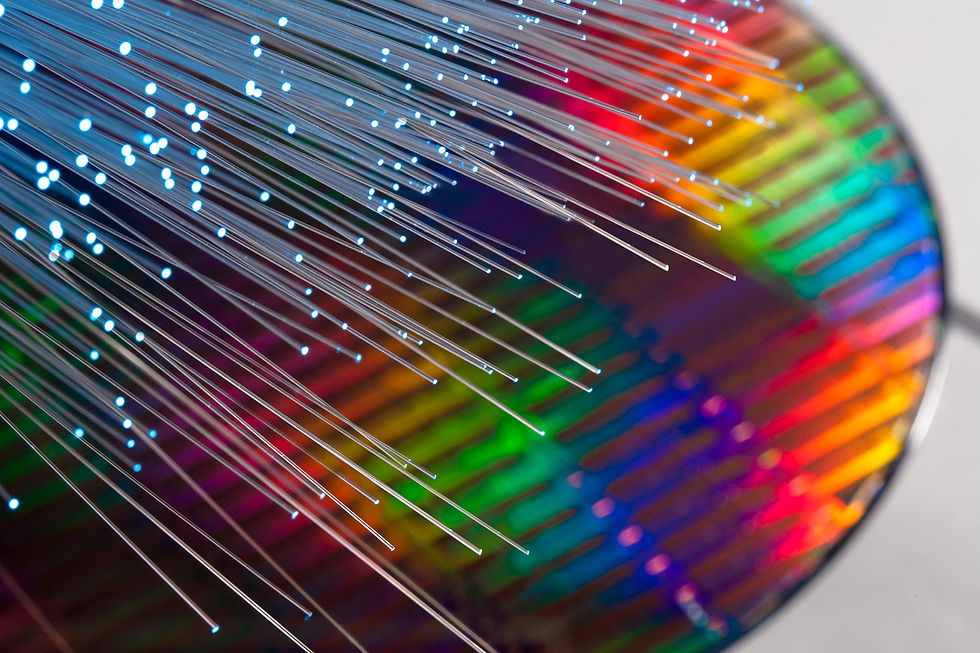
Photonics (encompassing both optics and optoelectronics) – is an emerging photon-based tech with the potential to yield non-biological neuromorphic neurons and neural networks by devising an all-optical neurosynaptic system. Implemented as an all-optical spiking neural network – closely imitating natural neural networks by incorporating time, firing only when a membrane potential reaches a specific value – on a nanophotonic chip, photonic neurosynaptic networks consist of supervised and unsupervised learning, high speed, high bandwidth, and direct processing of optical telco and visual data. A model of a previously proposed Superconducting Optoelectronic Network (SOEN) has many features of neural information processing. Superconducting Optoelectronic Network is a novel hardware platform for neuromorphic computing based on superconducting.
Spiking Neural Networks (SNNs) are computationally more powerful than other artificial neural network models regarding the number of neurons required. While biological information processing’s massively parallel communications and processing of sparse asynchronous binary signals motivate SNNs, neuromorphic hardware-based SNNs display beneficial properties. They include low power consumption, fast inference, and event-driven info processing. It makes them interesting candidates for the efficient implementation of deep neural networks, suitable for many machine learning tasks.

Spintronics is the study of the intrinsic spin of the electron and its associated magnetic moment in solid-state devices. Spin-orbit torque switching dynamics in antiferromagnet/ferromagnet heterostructures have shown the material system’s ability to form artificial neurons and synapses for asynchronous spiking neural networks. It opens a path toward executing cognitive tasks with the human brain efficiency with spintronics-based neuromorphic hardware.
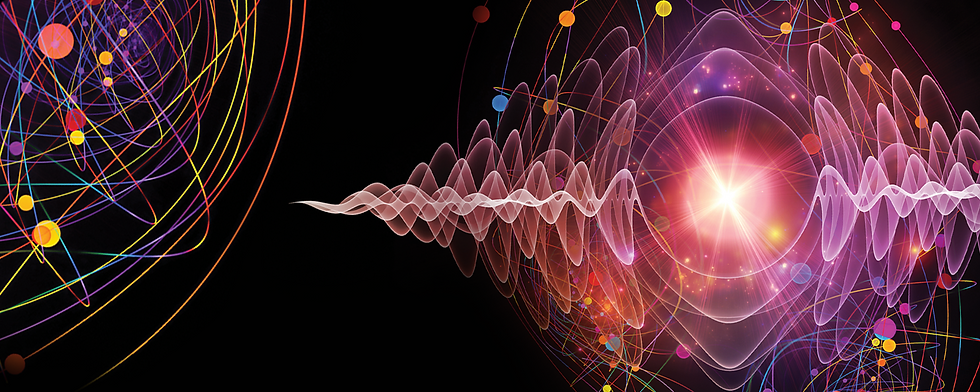
Quantum biology points to the emergence of bio-inspired quantum nanotechnologies that can operate in noisy room temp surroundings. These envisioned devices – their descriptor – perhaps merging into a de novo portmanteau including bioquantechnology - may enhance future neuromorphic AGI/post-AGI ecosystems with human neural structure & function.
Quantum stochasticity is both physical and application-based. There is a path where quantum events might influence brain activities. Universal wisdom holds that quantum fluctuations in macroscopic objects are inconsequential due to self-averaging – but with nonlinearly complex systems. It may be misleading: in chaotic systems, due to high sensitivity to initial conditions, we may amplify microscopic fluctuations upward and, therefore, affect the system’s output. Stochastic quantum dynamics might alter the outcome of many possible solutions thereby. Biological evolution can take advantage of quantum computational acceleration.
The Resistive Random Access (RRAM) conducive to filaments’ random information demonstrates a wide-ranging distribution. The RRDM MD self-assembled lead sulphide (PbS) quantum dots (QDs) improve RRAM uniformity of switching parameters in a process relatively straightforward compared to alternatives. They can offer a new way of improving memristor performance, can significantly expand existing applications and facilitate the development of artificial neural systems, can expand existing applications and facilitate the development of artificial neural systems.
We do not require neuromorphically identical substrates to establish isomorphic neural functionality between neural substrate variants. Neuromorphic solutions can be specific hardware independent or semi-independent.
Synthetic genomics may provide a biological route to an augmented neural environment. The most current dramatic illustration of synthetic genomics’ capabilities is the 2010 creation of an artificial cell.
Transdisciplinarity merges discrete scientific and technological domains and transcends traditional boundaries to synthesise de novo conceptual, theoretical, methodological, and translational innovations.
3. Future of AGI
According to S. MasonDambrot, the most powerful concept is Quantum Computing Artificial Superintelligence (qASI) variant. When such an environment achieves normalcy, the number of experienced physical and virtual exoselves (vision of the extended human in the future) could become prevalent. If sudden disentanglement by quantum decoherence unpredictably interrupts these quantum links, the human and AGI/ASI (Artificial General Intelligence/Artificial Superintelligence Intelligence) sense of loss may cause the bioself to experience a psychological response analogous to diminished cognitive function, memory loss, sensory deprivation, and/or a disorienting sense of loss and isolation. We need to take into account the mesh network interruption. We need to identify, address and prevent potential causes. Limiting exoself technology availability would create a deprived population unable to participate in exoself benefits. H. sapiens will accelerate the current transmutation first into what might be the Transhuman H. sapiens technologia subspecies.
In the context of the assumed futures envisioned above, the utility of automatic universal translation would be necessary. Fortunately, the likelihood of these scenarios' emergence is also feasible, given three interdigitated forms of neural enplants (endogenous implants). It is partly because of the possibility that people wishing or needing to use these technologies may have dramatically different physiologies, modes of communication and socio-cultural parameters.
A neural decoder explicitly leverages kinematic and sound representations encoded in human cortical activity to synthesise audible speech. It can provide a voice to those unable to communicate verbally due to neurological disabilities or others.
Ben Goertzel suggests that creating generally intelligent systems requires fundamentally distinct concepts and approaches than creating more specialised, “narrow AI” systems. To move the AGI field rapidly forward, one of two things must happen:
The emergence of a broadly accepted theory of general intelligence.
The demonstration of an AGI system that qualitatively seemingly demonstrates an impressive and considerable amount of general intelligence.
Comentários